1/ What are the challenges of AI? (Economy & public services) and its success factors? (Financing, PPP, research, HR, data & calculator, lab to market,)
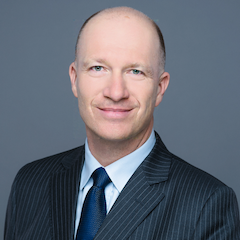
Renaud Vedel (RV): Over the past five years, there has been evidence that AI has the potential to become a “general purpose technology” as growth economists say, that is, a group of technologies that can transform not only the sciences but the entire productive system by profoundly renewing economic models. AI has already generated a number of innovations and hence industrial applications. As proof, the number of articles published on AI has multiplied by 6 in five years. Since 2011, the European Patent Office has seen a very strong growth in AI-based technologies in protected innovations. Thus, AI technologies or those related to them, such as data science, represent 12% of the total patents filed. At the same time, AI investment has increased fivefold from 4% to 21% of total venture capital investment in OECD countries. The challenge for Europe now is to disseminate these innovations in their economies and administrations in order to increase productivity, improve service and position themselves at the global technological frontier.
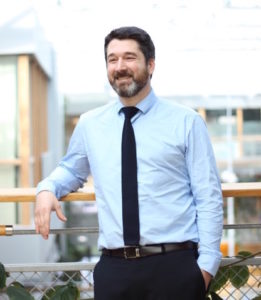
Julien Chiaroni (JC): The AI market is estimated at around €230 billion in 2020, with growth of almost 20% per year by 2024, driven in particular by its adoption in the industry. AI impacts, through the provision of new functionalities, all value chains, as much the development of new products and services as the improvement of production or control processes; this for many strategic industrial sectors for Europe. To take advantage of these opportunities offered by AI, three elements seem essential. First, we need to have a variety of skills. Investment in training is therefore essential. Second, build on competitive, interdisciplinary and multi-sectoral ecosystems at the national and European level. In a context of fierce international competition, the “collective” is fundamental. Third, mobilize significant investments to support the emergence of new markets, new players, but also strategic digital infrastructures in many industrial sectors. These digital infrastructures are key to digital sovereignty and the dissemination of European values.
RV: Keep in mind that AI is both a dynamic research field and an application field. AI research continues to explore the fundamentals of mathematics, the transition from cloud to embedded, how to represent knowledge, the quest for more frugality. At the same time, we must tackle the industrialization and economic potential of technologies that have already been developed. However, conversion to industrial uses, the lab to market, comes up against a shortage of skills, whether they are specialists at the PhD level or intermediate profiles. This is probably the main barrier for all countries, and this is why France has flagged half of the public funding dedicated to the AI of the investment plan «France 2030» towards training, with nearly 750 million euros dedicated to the skills needed for AI – from secondary to doctoral – out of the 2.2 billion in the Acceleration Strategy. Beyond human talents, machine learning requires large databases whose legal framework must be adapted to the learning function, which is different from the use of already developed models. The development of AI is conditioned by the fact of having, in each sector or organization, a policy of valorization and capitalization of data. This is essential in a world where the market for digital services and products is global, and where there is a strong premium on first-time entrants via network effects. The analogy too often made of the value of data with oil is therefore to be qualified: the data have much value until the first entrants in a market have developed their products, after which this value rapidly declines, the effects of networks playing to the full. The speed of time-to-market is therefore crucial.
JC: AI is indeed a general-purpose technology. It seems to me, however, that it carries an intrinsic specificity, both technical capacity and function of use. If I may use an example to illustrate my point, an autonomous vehicle uses many general-purpose technologies that are essential to its development, be they electronics or the cloud, but the use function is provided by AI. Based on this observation, two elements seem to me key: first, our ability to integrate a set of digital technologies (data, algorithms, electronics, cyber security, etc.), and therefore to carry a «system» and not only «algorithmic» vision or focused on “data”; second, our ability to cooperate within interdisciplinary and multisectoral ecosystems, between research and industry, large-scale groups and start-ups, etc. Finally, it is essential to reconcile “business” valuation AI in applications and the construction of digital infrastructures that play the role of enablers and therefore strategic in terms of digital sovereignty and economic competitiveness.
2/ The new legislation in progress, brake or booster?
JC: First of all, I would like to point out that trust is essential to the acceptability by users and citizens of AI technologies, and therefore to their dissemination in future products and services. The European regulatory proposal is thus a response to this fundamental challenge, but also to the dissemination of our vision of AI and the European values that we collectively carry. The question you are asking us is therefore a major one, especially since the European Commission has set out its ambition for a «horizontal» approach for a «generic» technology thus addressing many markets and industrial sectors. However, we must remain vigilant on two points that seem fundamental to me. First, we need to maintain an “acceptable” balance between regulation and innovation, while at the same time avoiding a “flood” of standards. Second, we need to ensure that there is an effective articulation between what is “generic” and what is specific industrial sectors. But I also see two major opportunities. It is an opportunity for Europe to innovate especially with regard to future solutions to ensure trust in AI-based systems. Above all, it is a real opportunity to develop a global benchmark digital infrastructure on AI confidence, addressing the issues of sovereignty and economic competitiveness mentioned above. To do this, we need a proactive industrial strategy on trusted AI that accompanies future AI regulation.
RV: Many observers consider that the European digital market is still far from being unified, and the EU’s first challenge is to remedy it. The maturity of the AI market in Europe is key for our leading start-ups to choose to stay on our continent rather than migrate to North America. From this point of view, the package of common legal rules proposed by the Commission on data and AI is an excellent thing. A second challenge will be to take into account simultaneously and with balance the risks and promises of these new technologies. The statistical nature of many contemporary AI systems, usable in a context of uncertainty, opens up new fields of automation and hence productivity. But AI must not enter into algorithmic human governance and remain respectful of rights and freedoms. The proposed horizontal legislation provides vision and imposes obligations on stakeholders to address the risks of robustness, bias and understanding of how high-risk AI systems work throughout their life cycle. Nevertheless, regulation is now faced with very rapid cycles of innovation that are not always used to regulations, both European and national. It is therefore necessary to provide flexibilities and spaces allowing technological creativity especially in the form of ambitious experiment sandboxes. Like the rest of digital, innovation often follows iterative paths in a market situation. This calls for a major shift towards sectoral technical standardisation and the invention of new ways of adapting regulations in short cycles.
3/ How to develop AI systems in a sustainable way? (Public & private examples)
JC: The term “sustainable” is key to your question. First, the sustainability of AI systems refers to their energy and environmental footprints. It is unthinkable to continue the exponential trend of the energy cost of AI. Frugality, both in terms of data and the power and computational time required, thus requires a major effort of research, development and industrialization. In this regard, colleagues are interested in the implementation of a shared methodology for assessing the energy and environmental impact of AI. Second, a sustainable system requires that it be industrialized and deployed on one or more markets. Outside, this is a major issue because only 10 to 15% of proof of concept (POC, proof of concept) in AI are industrialised and scale. And one of the main obstacles to this industrialization is “trust”, respect for requirements of safety, responsibility or ethics, Hence again the importance of a proactive industrial strategy in relation to the future European regulation in AI.
RV: The sustainable development of AI involves both hardware and algorithms. The industry is working on the development of AI-enabled electronic components and computational architectures. Artificial intelligence is therefore one of the dimensions of the EU’s strategic autonomy and resilience in the electronic sector. In particular, the emerging field of embedded AI needs to be addressed and the French strategy emphasizes this issue. It is also necessary to take into account the longer-term challenge of controlling, opening and sharing data for AI, fighting monopolies and defending the portability of data through rules of the game applicable to all. The development of digital commons in embedded AI can make it possible to regain more sovereignty over these segments. Finally, on the environmental front, it is still difficult to decide. There is now a kind of race to gigantism of models whose efficiency and not only apparent effectiveness must be assessed. There is a need to develop metrics of energy consumption compared to potentially usable AI systems for each application. The idea that we can put AI everywhere is therefore probably not sustainable today, and we will have to choose the use cases with the highest collective added value to ensure net gains from the point of view of ecological transition.
4/ What should the «4th industrial revolution» bring us? (Resources, employment, well-being…)
RV: The fourth industrial revolution contains four types of promises. It is first of all a leap in scientific knowledge: by allowing to process information of great complexity, the AI applied to large masses of data has allowed a leap forward in the understanding of proteins, in climate modelling, and in a number of other scientific fields today very dynamic. The second promise is greater personalization of services. This is particularly the case in education with new perspectives in the personalization of learning as well as in the medical field, for example in the personalization of diagnosis, prognosis and therapy. The third promise concerns the automation of difficult tasks, whether physical or cognitive. Finally, the 4th industrial revolution should make it possible, via «digital twins» and computational efficiency, to have production systems much more optimized and therefore much more sober, particularly in areas seeking to reduce their energy footprint or their inputs such as agriculture. If we manage to focus AI on these four issues, the 4th Industrial Revolution will bring more positive than negative externalities.
JC: Of course, I share Renaud’s four promises. So I’m going to focus on highlighting the challenges. For example, personalization questions privacy, automation about the future of work and employment, the production of knowledge about its sharing and training. We must collectively reflect on these challenges.